Research Interest
-
Hydrology and Water Resources
-
Hydroclimatic Extremes
-
Compound Extremes
-
Climate Adaptation and Resilience
-
Global Change and Sustainability
-
Remote Sensing Applications
-
Environmental Informatics
Research Project
-
PI, Elucidating the Mechanisms and Dynamics of Flash Drought from the Perspective of Land‒Atmosphere Feedbacks, Hong Kong Research Grants Council General Research Fund, 2024–2026.
-
PI, Drought Hazard Assessment and Projection in A Warming Climate, FCE Young Researcher Collaborative Research Fund, 2023–2025.
-
PI, Incorporating Spatial Heterogeneity of Rainfall Response to Climate Change into the Design of Slope Drainage Provisions in Hong Kong, Public Policy Research (PPR) Funding Scheme, 2023–2024.
-
PI, Climate-Resilient Planning and Design for Coastal Stormwater Drainage Systems, Environment and Conservation Fund (ECF), 2022–2024.
-
PI, Integration of Emerging Drought Risk into Hong Kong’s Climate Adaptation Planning and Environmental Policy, RILS Strategic Supporting Scheme, 2022–2024.
-
PI, Urban Flood Risk Assessment and Prediction Under Climate Change, Otto Poon Charitable Foundation Smart Cities Research Institute (SCRI) Fund, 2022–2023.
-
PI, Detection and Projection of Coastal Erosion Hotspots Driven by Extreme Sea Levels under a Changing Climate, RILS Strategic Supporting Scheme, 2021–2023.
-
PI, Integration of InSAR, GNSS, and Earth System Modeling Techniques for Predicting Future Change in Coastal Inundation Risk, LSGI Collaborative Research Grant, 2021–2023.
-
PI, Improving Robustness and Reliability of Hydroclimatic Projections through A New Multi-Model Ensemble Framework, Hong Kong Research Grants Council Early Career Scheme, 2020–2022.
-
PI, A Stochastic Multi-Model Ensemble Framework for Probabilistic Hydrological Forecasting, National Science Foundation of China, 2019–2021.
-
PI, Improving Urban Resilience to Projected Changes of Extreme Weather Events, Hong Kong Polytechnic University Start-up Fund, 2017–2020.
Media Coverage
-
Drought:
Nature, ScienceDaily, Phys.org, Grist, ScienMag, Mirage News, Iowa Climate, PolyU, PolyU Pulse Newsletter,
UT Austin, TEXAS Global, etc.
新浪, 搜狐, 知乎, 騰訊新聞, 中國新聞網, 鳳凰新聞, 點新聞, 環球網, 新聞傳媒網, 中國氣象局, 頭條日報, 星島日報, 晴報,
香港新聞, 壹讀, 東網, 巴士的報, 東方日報, etc.
-
Drought-to-Downpour Shift:
Yahoo UK, Mirage News, Earth.com, The Daily Texan, Phys.org, WTX News, Newsweek, Euronews, TechNews, PolyU PAIR,
UT Austin, etc.
-
Heatwaves and Extreme Sea Levels:
The United Nations Office for Disaster Risk Reduction (UNDRR), Scientific American, Nature Asia, France 24, Scimex, Yahoo UK,
Phys.org, CCTV, Xinhua Net, People's Daily, China News, Tencent News, PolyU PAIR, etc.
Research Work
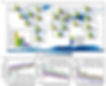

Hydroclimatic Extreme
Selected Research Outputs:
-
Qing, Y., Wang, S., Yang, Z-L., and Gentine, P. (2023) Soil moisture−atmosphere feedbacks have triggered the shifts from drought to pluvial conditions since 1980. Communications Earth & Environment, 4, 254.
-
Qing, Y. and Wang, S., Ancell, B., and Yang, Z-L. (2022) Accelerating flash droughts induced by the joint influence of soil moisture depletion and atmospheric aridity. Nature Communications, 13, 1139.
-
Li, X. and Wang, S. (2022) Recent increase in the occurrence of snow droughts followed by extreme heatwaves in a warmer world. Geophysical Research Letters, 49, e2022GL099925.
-
Chen, H. and Wang, S. (2022) Accelerated transition between dry and wet periods in a warming climate. Geophysical Research Letters, 49, e2022GL099766.
-
You, J. and Wang, S. (2021) Higher probability of occurrence of hotter and shorter heat waves followed by heavy rainfall. Geophysical Research Letters, 48, e2021GL094831.



Hydroclimatic Prediction
Selected Research Outputs:
-
Zhang, B., Wang, S., Moradkhani, H., Slater, L., and Liu, J. (2022) A vine copula-based ensemble projection of precipitation intensity-duration-frequency curves at sub-daily to multi-day time scales. Water Resources Research, 58, e2022WR032658.
-
Zhang, B., Wang, S., Qing, Y., Zhu, J., Wang, D., and Liu, J. (2022) A vine copula-based polynomial chaos framework for improving multi-model hydroclimatic projections at a multi-decadal convection-permitting scale. Water Resources Research, 58, e2022WR031954.
-
Zhang, B., Wang, S., and Wang, Y. (2021) Probabilistic projections of multidimensional flood risks at a convection-permitting scale. Water Resources Research, 57, e2020WR028582.
-
Zhang, B., Wang, S., and Wang, Y. (2019) Copula‐based convection‐permitting projections of future changes in multivariate drought characteristics. Journal of Geophysical Research: Atmospheres, 124, 7460–7483.
-
Wang, S., Ancell, B.C., Huang, G.H., and Baetz, B.W. (2018) Improving robustness of hydrologic ensemble predictions through probabilistic pre- and post-processing in sequential data assimilation. Water Resources Research, 54, 2129–2151.
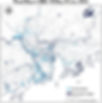
Coastal Hazard and Risk Assessment
Selected Research Outputs:
-
Zhou, M. and Wang, S. (2024) The risk of concurrent heatwaves and extreme sea levels along the global coastline is increasing. Communications Earth & Environment, 5, 144.
-
Zhang, B. and Wang, S. (2021) Probabilistic characterization of extreme storm surges induced by tropical cyclones. Journal of Geophysical Research: Atmospheres, 126, e2020JD033557.
-
Carvalho, K.S. and Wang, S. (2019) Characterizing the Indian Ocean sea level changes and potential coastal flooding impacts. Journal of Hydrology, 569, 373–386.
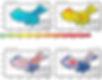
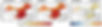
Heat Stress and Temperature Extreme
Selected Research Outputs:
-
You, J., Wang, S., Zhang, B., Raymond, C., and Matthews, T. (2023) Growing threats from swings between hot and wet extremes in a warmer world. Geophysical Research Letters, 50, e2023GL104075.
-
Zhu, J., Wang, S., and Fischer E.M. (2022) Increased occurrence of day-night hot extremes in a warming climate. Climate Dynamics, 59, 1297–1307.
-
Zhu, J., Wang, S., Zhang, B., and Wang, D. (2021) Adapting to changing labor productivity as a result of intensified heat stress in a changing climate. GeoHealth, 4, e2020GH000313.
-
Wang, S. and Zhu, J. (2020) Amplified or exaggerated changes in perceived temperature extremes under global warming. Climate Dynamics, 54, 117–127.
-
Zhu, J., Wang, S., and Huang, G. (2019) Assessing climate change impacts on human-perceived temperature extremes and underlying uncertainties. Journal of Geophysical Research: Atmospheres, 124, 3800–3821.